Opinions expressed on this site are solely those of Kendra Little of Catalyze SQL, LLC. Content policy: Short excerpts of blog posts (3 sentences) may be republished, but longer excerpts and artwork cannot be shared without explicit permission.
By Kendra Little on April 13, 2025
Statistics in SQL Server are simple in theory: they help the optimizer estimate how many rows a query might return.
In practice? Things get weird fast. Especially when you start filtering on multiple columns, or wondering why the optimizer thinks millions of rows are coming back when you know it’s more like a few hundred thousand.
In this post, I’ll walk through examples using single-column, multi-column, and filtered statistics—and show where estimates go off the rails, when they get back on track, and why that doesn’t always mean you need to update everything with FULLSCAN.
I’m using the StackOverflow2013 sample database at compat level 160
To get started, I’ve dropped all my existing indexes and statistics. I’ve created a helper object that lists all the statistics on a given table.
alter database StackOverflow2013 SET COMPATIBILITY_LEVEL=160;
GO
use StackOverflow2013
GO
CREATE OR ALTER PROC dbo.ListStatistics
@ObjectName SYSNAME
AS
SET NOCOUNT ON;
SELECT
s.name AS StatisticName,
c.name AS LeadingColumn,
s.auto_created,
s.user_created,
s.has_filter,
s.filter_definition,
s.no_recompute
FROM sys.stats AS s
JOIN sys.stats_columns AS sc
ON s.object_id = sc.object_id AND s.stats_id = sc.stats_id
JOIN sys.columns AS c
ON sc.object_id = c.object_id AND sc.column_id = c.column_id
WHERE sc.stats_column_id = 1
AND s.object_id = OBJECT_ID(@ObjectName)
ORDER BY s.name;
GO
Before I get started, the dbo.Posts
table has a single statistic.
This statistic is associated with the clustered index on the Id
column. Whenever an index is created in SQL Server, a statistic is created along with that index.

Run our example query, which will auto-create single column statistics to help with estimates.
Our example query has three predicates, on PostTypeId
, ParentId
, and AnswerCount
.
To simplify things so auto-parameterization and query plan reuse don’t involved, I’ve added an OPTION (RECOMPILE)
hint to the query.
SELECT Id
FROM dbo.Posts
WHERE PostTypeId=1
and ParentId = 0
AND AnswerCount = 0
OPTION (RECOMPILE);
When I give this a run, the query plan shows…
- SQL Server estimates that 3,200,270 rows will be returned.
- 305,761 rows are actually returned– only 9.5% of what was estimated. That estimate was very high.
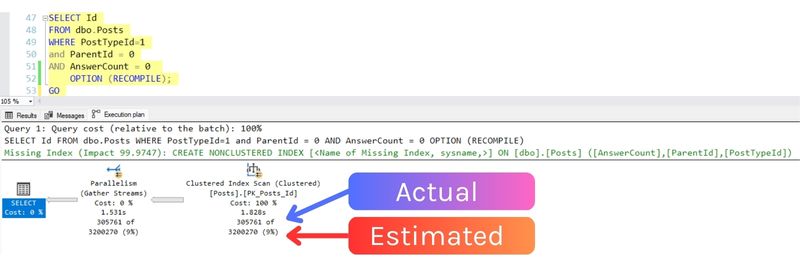
I re-run dbo.ListStatistics
and see that three column statistics were automatically created, one on each of the three predicates in my query. I have the AUTO_CREATE_STATISTICS
setting enabled for the StackOverflow2013 database– that’s a default setting, and it allows SQL Server to create these small helper objects to put together this estimate.
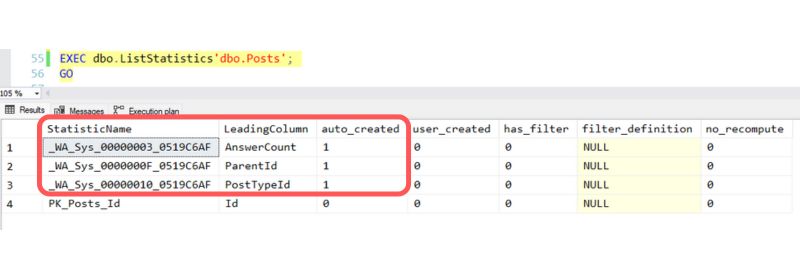
Each of these statistics took a sampling of rows from the table and used that sampling to create a histogram with “steps” describing what values are in the table and how many rows exist for some values. This is stored along with metadata: how many rows were in the table at the time of the sampling? How many rows were sampled? About how many unique values exist in the column?
For example, here is a view of the histogram for the automatically created single column statistic on the PostTypeId column:
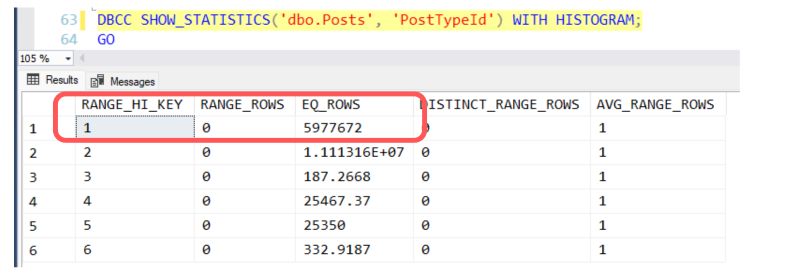
Based on a sampling of the data, there were estimated to be 5,977,672 rows in dbo.Posts with PostTypeId=1 at the time the statistic was auto-created.
The column statistics created for ParentId and AnswerCount similarly describe how data is distributed in those columns.
SQL Server can use these statistics to make estimates, but note that none of these statistics describe data distributions for all three columns when they are used together.
When we create an index with multiple key columns, it creates a multi-column statistic to go with it
Let’s say we have an index on the first two columns in our predicate. Here’s the index:
CREATE INDEX ix_Posts_PostTypeId_ParentId
ON dbo.Posts (PostTypeId, ParentId)
WITH (DATA_COMPRESSION=PAGE);
GO
I’ve created a nonclustered index that does not fully “cover” my query: it has two out of three predicates.
I run my query again, and SQL Server does not choose to use my new index. Its row estimate is slightly different:
- Before, SQL Server had estimated 3,200,270 rows would be returned.
- Now, SQL Server estimates 3,212,350 rows will be returned (12,080 more)
- This estimate is still very high, only 305,761 rows are returned (the same, rows in the database are not changing)
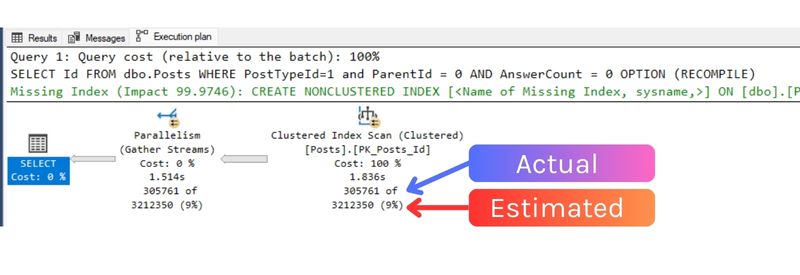
Why is this estimate just a little bit higher?
If I use DBCC SHOW_STATISTICS
to describe the statistic associated with the index, it does describe a little bit about more than the first column, but not much. Important things to notice are:
- This statistic was created when SQL Server created the index, and it had to look at every row in the column to do that. It used that information from all the rows (rather than a sampling), so the data in the statistic is a little different.
- The statistic has a “Density vector” section, which describes the average selectivity of column values—in other words, how distinct the values tend to be.
- It gives the inverse of the number of distinct (PostTypeId, ParentId) pairs.
- For example, if the all density value is 1.779737E-07, that implies there are about 5.6 million distinct (PostTypeId, ParentId) combinations (1/.0000001779737= 5,618,807).
- There are 1,7142,169 rows in the table, which implies that any given PostTypeId, ParentId column has about 3.05 rows for it IF there is a very even distribution of values.
- The histogram of the statistic is only on the leading column in the index, which in this case is PostTypeId. PostTypeId has a pretty limited set of values.
- The histogram shows 6,000,223 values for PostTypeId = 1. In the column statistic on PostTypeId that was based on sampling, this estimate was 5,977,672. The estimate has gone up by 22,551.
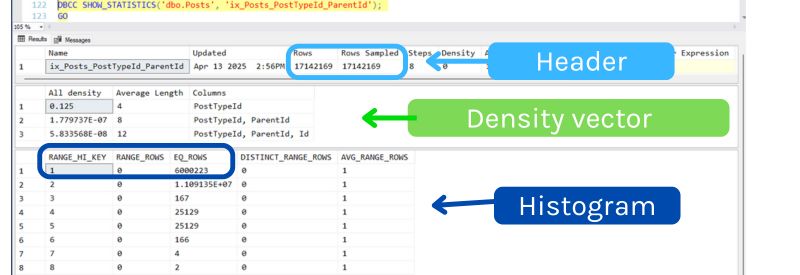
In this case, it looks like the fact that we have a multi-column statistic didn’t really give the optimizer too much new information it thought was useful.
Yes, the density vector implies that there are 3.05 rows on average for any given combination of PostTypeId and ParentId values on average, but the estimate is way higher than 3 rows. It looks like our estimate is still based on histogram values, and the estimate went up a little bit because the histogram for the new index– which is only on the leading column, PostTypeId– estimated a slightly higher number of rows for PostTypeId = 1.
Create a multi-column filtered statistic tailored to the query
Now, I could create a multi-column filtered index that is perfect for my query. But let’s say that this query doesn’t run that often, and I don’t want to create an index just for it. However, I want to see if SQL Server might be smarter if I gave it a statistic.
I create a statistic that is very targeted for my query, like this:
CREATE STATISTICS [FilteredStats_Posts_PostTypeId_ParentId_AnswerCount]
ON dbo.Posts (PostTypeId, ParentId, AnswerCount)
WHERE PostTypeId = 1 AND ParentId = 0 AND AnswerCount = 0;
GO
One thing I’ve noticed is that when you create a statistic like this, it won’t automatically make queries recompile. I have an OPTION (RECOMPILE)
clause in my query, though, so I don’t have to worry about that.
Rerunning my query, I get the same shape plan, but the estimates are much more accurate:
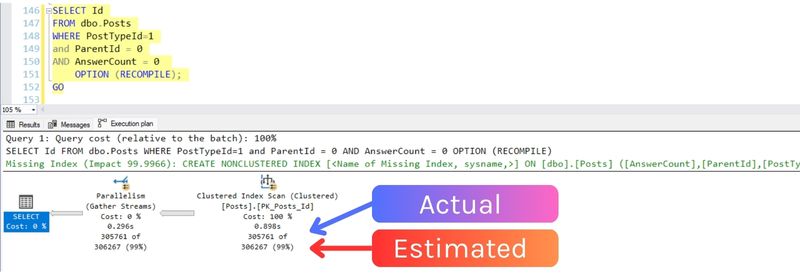
With the filtered multi-column statistic, SQL Server is estimating that 306,267 will be returned, very close to the actual rowcount of 305,761.
This doesn’t make much of a difference in this simple case, but if I was joining to this table and the data was flowing into an operator that needed to allocate memory, like a hash join or a sort, this estimate could make a difference.
Real world talk: I don’t go around creating statistics like this “just in case”, and I wouldn’t assume that this will automatically solve a problem– but sometimes this can help in a specific situation where it doesn’t make sense to create an index and poor row estimates are causing a performance problem.
The query plan depends on which version of the cardinality estimator (CE) I’m using
SQL Server has two cardinality estimators: the “legacy” cardinality estimator, which applies to database compatibility level 110 and below (or if you have it enabled as a database scoped configuration), and the “doesn’t really have a name”/newer cardinality estimator.
The legacy cardinality estimator is more likely to assume that data distributions on different columns are independent of one another.
If I have the filtered statistic created and the two column index created, and I run my query using the legacy cardinality estimator, SQL Server decides to use the non-covering index and do a nested loop lookup to pick up the AnswerCount data (which is not in the index).
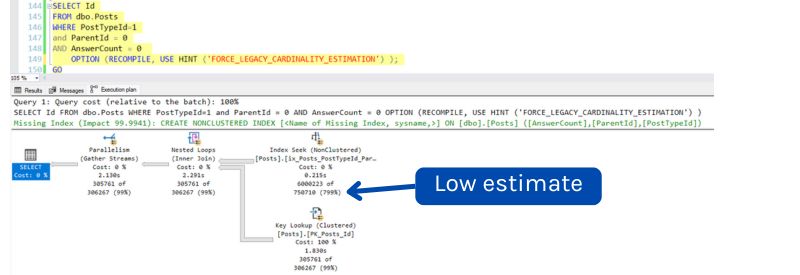
The row estimate for how much will come out of the nested loop operator is very accurate: 306,267 rows (actual is 305,761).
However, SQL Server estimates that 750,710 rows will come out of the index on PostTypeId
, ParentId
, whereas it actually had 6,000,223 rows returned from the operator.
Tips and takeaways about statistics
-
Statistics help SQL Server guess row counts.
-
Statistics are estimates, and close-enough is usually fine. Don’t chase perfection.
-
Auto-created single-column stats have a histogram that describes how data is distributed in one column at a time. If your query filters on multiple columns, SQL Server has to guess how those filters work together.
-
Multi-column stats (created with multi-column indexes, or manually) only have a histogram for the leading column.
-
Filtered statistics can help with tricky queries where the estimate is way off and you don’t want (or need) a new index. If you match the filter exactly, the estimate can get much better.
-
Don’t assume “bad estimate” means “bad stat” or “bad plan”. Estimates can be off and still lead to a good plan. Make sure to verify if your problem has to do with “statistics were wrong” or if it’s a case of “the statistics were fine for the values the query plan was compiled with, but then the plan ran with different values for parameters” (a parameter sniffing problem).
-
Avoid the “just fullscan all your statistics” trap. Updating statistics with fullscan all the time burns IO and slows down over time. Becoming dependent upon this can leave you in a reactive position where you regularly have poor performance.
-
The version of the cardinality estimator matters. Sometimes the legacy cardinality will work better for a query. Sometimes it won’t.